What is tool calling?
LLM tool calling allows a language model (like GPT) to use external tools (functions inside your codebase) to perform tasks it can't handle alone.
Instead of just generating text, the model can respond with a tool call (name of the function to call with parameters) that triggers a function in your code.
You can use tool calling to get the model to do things like fetch live information, run code for complex calculations, call another get some data from a database, call another pipe, or interact with other systems.
How does it work?
Langbase offers tool calling with multiple providers (view complete list) to provide more flexibility and control over the conversation. You can use this feature to call tools in your code and pass the results back to the model.
- Describe the tool: You can describe a tool in your Pipe by providing the tool name, description, and arguments. You can also specify the data type of the arguments and if they are required or optional. These tools are then passed to the model.
- User prompt: You sent a user prompt that requires data that the tool can provide. The model will generate a JSON object with tool name and its arguments.
- Call the tool: You can use this JSON object to call the tool in your code.
- Pass the result: You can pass the result back to the model to continue the conversation.
Tool definition schema
Tool calling features requires you to add a tool definition schema in your Pipe. This tool definition is passed to the model. The model then generates a JSON object with the tool name and its arguments based on the user prompt.
Here is an example of a valid tool definition schema:
{
"type": "function",
"function": {
"name": "get_current_weather",
"description": "Get the current weather of a given location",
"parameters": {
"type": "object",
"required": [
"location"
],
"properties": {
"unit": {
"enum": [
"celsius",
"fahrenheit"
],
"type": "string"
},
"location": {
"type": "string",
"description": "The city and state, e.g. San Francisco, CA"
}
}
}
}
}
Prerequisites: Generate Langbase API Key
We will useLangbase SDK in this guide. To work with it, you need to generate an API key. Visit User/Org API key documentation page to learn more.
How to make tool calls in Pipes using Langbase SDK
Follow this quick guide to learn how to make tool calls in Pipes using Langbase SDK.
Step #0Create a Pipe
Create a new Pipe or open an existing Pipe in your Langbase account. Alternatively, you can fork this tool-calling-example
pipe and skip to step 2.
Step #1Add a tool to the Pipe
Let's add a tool to get the current weather of a given location. Click on the Add
button in the Tools section to add a new tool.
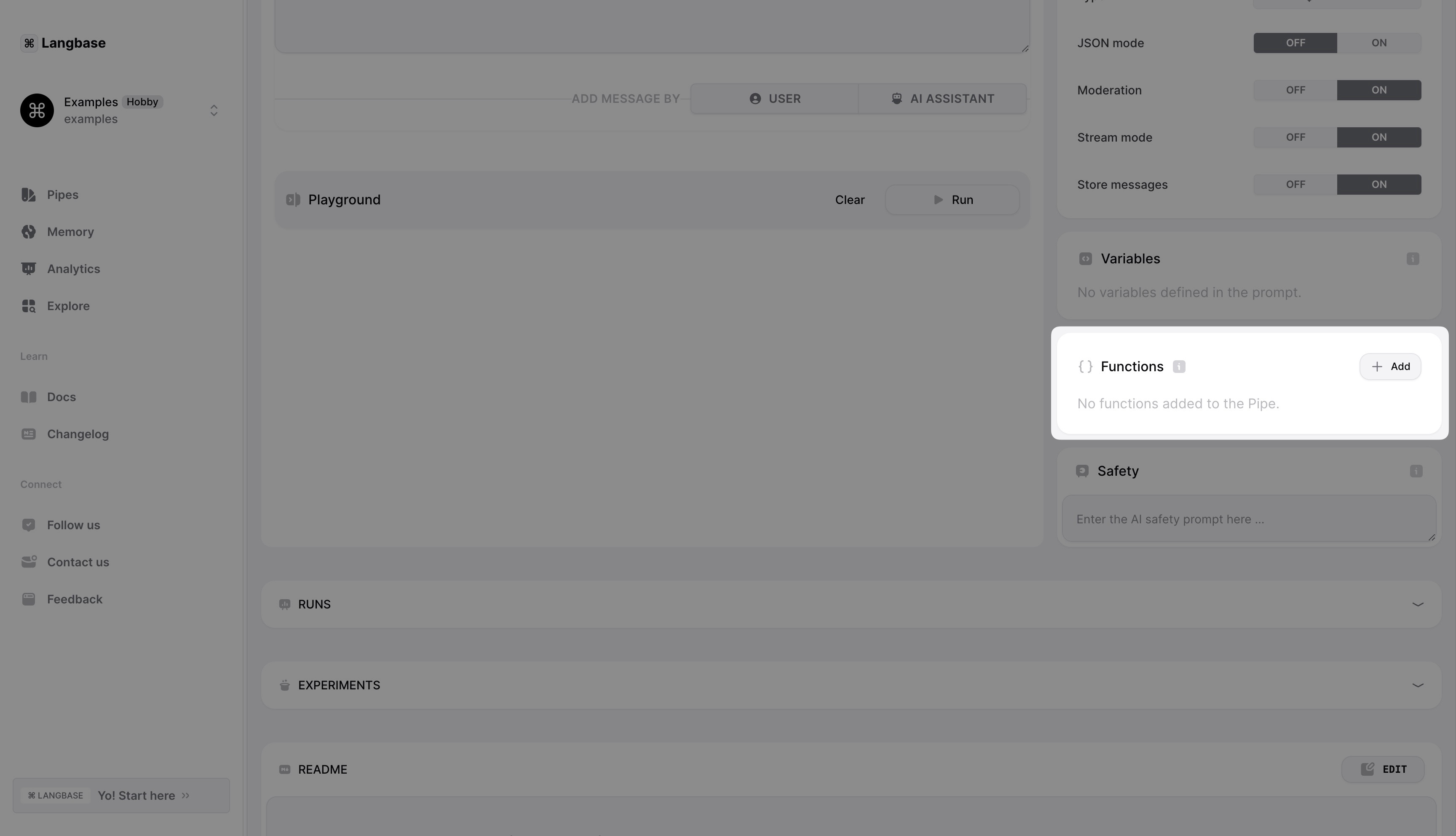
This will open a modal where you can define the tool. The tool we are defining will take two arguments:
- location
- unit.
The location
argument is required and the unit
argument is optional.
The tool definition will look something like the following.
{
"type": "function",
"function": {
"name": "get_current_weather",
"description": "Get the current weather of a given location",
"parameters": {
"type": "object",
"required": [
"location"
],
"properties": {
"unit": {
"enum": [
"celsius",
"fahrenheit"
],
"type": "string"
},
"location": {
"type": "string",
"description": "The city and state, e.g. San Francisco, CA"
}
}
}
}
}
Go ahead and deploy the Pipe to production.
Step #2Install dependencies
We will use Langbase SDK to run the pipe. We will also use dotenv
to load env variables from a .env
file.
Install the SDK
npm i langbase dotenv
Step #3Add env variables
Create a .env
file in the root of your project and add the following environment variables.
LANGBASE_API_KEY="<USER/ORG-API-KEY>"
Replace <USER/ORG-API-KEY>
with your Langbase API key.
Step #4Run pipe with user prompt to make a tool call
Now let's create an index.js
file where we will define get_current_weather
function and also run the pipe with a user prompt that will trigger the tool call.
import 'dotenv/config';
import { Langbase, getToolsFromStream, getRunner } from 'langbase';
const langbase = new Langbase({
apiKey: process.env.LANGBASE_API_KEY, // https://langbase.com/docs/api-reference/api-keys
});
function get_current_weather() {
return "It's 70 degrees and sunny in SF.";
}
const tools = {
get_current_weather,
};
async function main() {
const messages = [
{
role: 'user',
content: 'Whats the weather like in SF?',
},
];
const { stream: initialStream, threadId } = await langbase.pipes.run({
messages,
stream: true,
name: 'tool-calling-example', // name of the pipe to run
});
}
main();
Replace tool-calling-example
with your pipe name if it has a different name.
Here is what we did so far:
- Initialized the Langbase SDK with the API key.
- Defined a
get_current_weather
function that returns the current weather of San Francisco. - Created a
tools
object that contains theget_current_weather
function. - Wrote a
main
function that runs the Pipe using SDK with a user prompt that triggers the tool call.
Because the user prompt requires the current weather of San Francisco, the model will respond with a tool call like the following:
{
"role": "assistant",
"content": null,
"tool_calls": [
{
"id": "call_u28sPmmCAWkop0OdgDYDJ9OG",
"type": "function",
"function": {
"name": "get_current_weather",
"arguments": "{\"location\": \"San Francisco\"}"
}
}
]
}
Step #5Check for tool calls
We will still check if we recieved any tool calls from the model. If the model has called a tool, we will call the functions specified in the tool call and send the tool results back to model using Langbase SDK.
import 'dotenv/config';
import { Langbase, getToolsFromStream, getRunner } from 'langbase';
const langbase = new Langbase({
apiKey: process.env.LANGBASE_API_KEY, // https://langbase.com/docs/api-reference/api-keys
});
function get_current_weather() {
return "It's 70 degrees and sunny in SF.";
}
const tools = {
get_current_weather,
};
async function main() {
const messages = [
{
role: 'user',
content: 'Whats the weather like in SF?',
},
];
const { stream: initialStream, threadId } = await langbase.pipes.run({
messages,
name: 'tool-calling-example', // name of the pipe to run
stream: true,
});
const [streamForToolCalls, streamForResponse] = initialStream.tee();
const toolCalls = await getToolsFromStream(streamForToolCalls);
const hasToolCalls = toolCalls.length > 0;
if (hasToolCalls) {
const messages = [];
// call all the functions in the toolCalls array
toolCalls.forEach(async toolCall => {
const toolName = toolCall.function.name;
const toolParameters = JSON.parse(toolCall.function.arguments);
const toolFunction = tools[toolName];
const toolResult = toolFunction(toolParameters);
messages.push({
tool_call_id: toolCall.id, // required: id of the tool call
role: 'tool', // required: role of the message
name: toolName, // required: name of the tool
content: toolResult, // required: response of the tool
});
});
const { stream: chatStream } = await langbase.pipes.run({
messages,
name: 'tool-calling-example', // name of the pipe to run
threadId: threadId,
stream: true,
});
const runner = getRunner(chatStream);
runner.on('content', content => {
process.stdout.write(content);
});
} else {
// no tool calls, handle the response stream
}
}
main();
Lastly, here is what we did:
- Cloned the initial stream to use it later for tool calls check.
- Used
getToolsFromStream
SDK function to get tool calls from the stream if any. - Checked if there are any tool calls.
- Incase there are tool calls, we called the functions specified in the tool call.
- We then sent the tool results in a messages array to the model using Langbase SDK.
- We also sent the
threadId
in the request to the pipe to continue the conversation.
Step #6Run the code
Let's run the code using the following command:
node index.js
You should see the following output printed on your terminal:
The weather in San Francisco is 70 degrees Celsius and sunny.
And that's it! You have successfully made a tool call using pipes & Langbase SDK.
Next Steps
- Build something cool with Langbase.
- Join our Discord community for feedback, requests, and support.